A Systematic Literature Review on Multimodal Medical Image Fusion
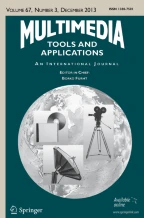
Medical image fusion is a relevant area with widespread application in disease diagnosis and prediction with easily available image scans of Computed Tomography, Positron Emission Tomography, Magnetic Resonance Imaging, and Single Photon Emission Computed Tomography. Each diagnostic image modality has its advantages and limitations. Multimodal Medical Image Fusion aims to combine more than one image of the same or different modality to enhance the image content and provide more information about diseases. We performed a Systematic Literature Review according to the methodology outlined in Kitchenham Charter and based on our search string, we extracted 844 studies from four electronic databases published between 2017 and 2021. Around 175 studies were selected for further in-depth analysis using inclusion and exclusion criteria. We further divide this review article into five sections that (a) Identify the most frequently used input image decomposition methods (b) Describes the most common fusion rules applied on decomposed sub-bands (c) Discusses the optimization algorithms used to improve the efficiency of the fusion scheme (d) Examines the modalities which are subjected to image fusion techniques in the medical domain (e) Identifies the evaluation metrics used to judge the effectiveness of image fusion technique. The result of the comparative study of five sections highlights that the majority of studies use multiscale decomposition methods, and hybrid and neural network-based fusion rules while the CT-MRI combination was mostly used as an input dataset. The review also indicated the prevalent use of particle swarm optimization and non-reference metrics in the majority of studies. Our results suggest that medical image fusion can improve the quality and accuracy of medical images for diagnosis and treatment planning. Further research can be conducted to handle potential research gaps outlined in this review and optimize medical image fusion for clinical applications.
This is a preview of subscription content, log in via an institution to check access.
Access this article
Subscribe and save
Springer+ Basic
€32.70 /Month
- Get 10 units per month
- Download Article/Chapter or eBook
- 1 Unit = 1 Article or 1 Chapter
- Cancel anytime
Buy Now
Price includes VAT (France)
Instant access to the full article PDF.
Rent this article via DeepDyve
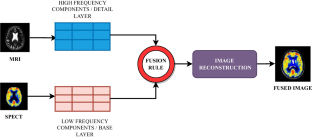
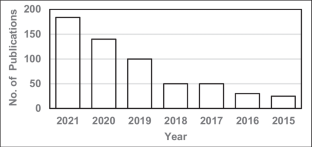
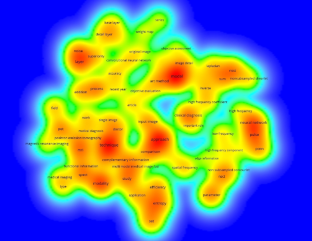

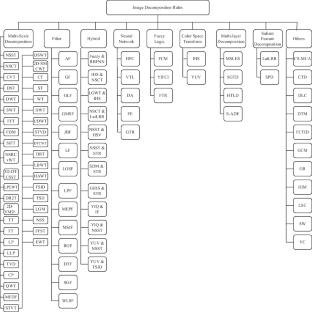
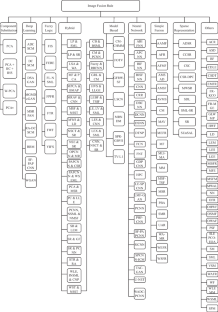
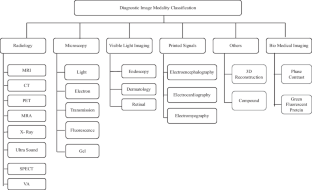
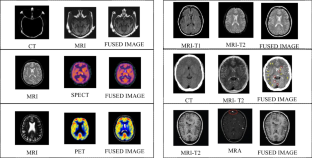
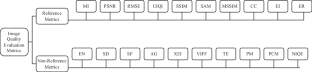
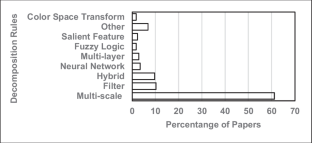
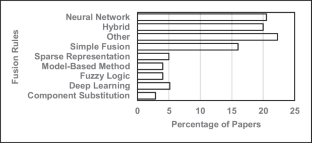
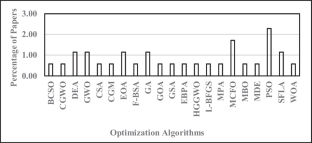
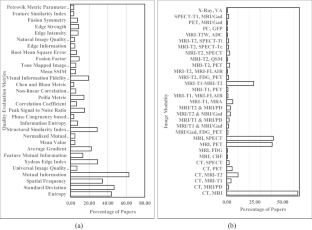
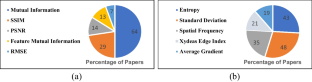
Similar content being viewed by others
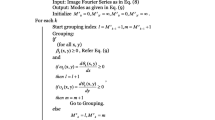
Structural and functional medical image fusion using an adaptive Fourier analysis
Article 11 June 2020
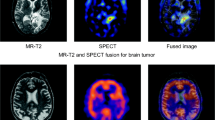
Survey study of multimodality medical image fusion methods
Article 15 October 2020
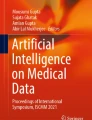
A Study on Various Medical Imaging Modalities and Image Fusion Methods
Chapter © 2023
Explore related subjects
- Medical Imaging
- Artificial Intelligence
Availability of data and materials
Data sharing not applicable to this article as no datasets were generated or analyzed during the current study
References
- Abas A, Kocer HE, Baykan N (2021) Medical image fusion with convolutional neural network in multiscale transform domain. Turk J Electr Eng Comput Sci 29(8):2780–2794 Google Scholar
- Aishwarya N, Bennila Thangammal C (2018) A novel multimodal medical image fusion using sparse representation and modified spatial frequency. Int J Imaging Syst Technol 28(3):175–185 Google Scholar
- Akbarpour T, Shamsi M, Daneshvar S, Pooreisa M (2019) Medical image fusion based on nonsubsampled shearlet transform and principal component averaging. Int J Wavelets, Multiresolution Inf Process 17(04):1950023 MathSciNetGoogle Scholar
- Anand RS, Singh S (2019) Multimodal neurological image fusion based on adaptive biological inspired neural model in nonsubsampled shearlet domain. International Journal of Imaging Systems and Technology 29(1):50–64 Google Scholar
- Arif M, Wang G (2020) Fast curvelet transform through genetic algorithm for multimodal medical image fusion. Soft Comput 24(3):1815–1836 Google Scholar
- Asha C, Lal S, Gurupur VP, Saxena PP (2019) Multi-modal medical image fusion with adaptive weighted combination of nsst bands using chaotic grey wolf optimization. IEEE Access 7:40782–40796 Google Scholar
- Azam MA, Khan KB, Ahmad M, Mazzara M (2021) Multimodal medical image registration and fusion for quality enhancement. Comput Mater Continua 68:821–840 Google Scholar
- Bengueddoudj A, Messali Z, Mosorov V (2017) A novel image fusion algorithm based on 2d scale-mixing complex wavelet transform and bayesian map estimation for multimodal medical images. J Innov Opt Health Sci 10(03):1750001 Google Scholar
- Bhardwaj J, Nayak A (2020) Haar wavelet transform-based optimal bayesian method for medical image fusion. Med Biol Eng Comput 58(10):2397–2411 Google Scholar
- Bhateja V, Srivastava A, Moin A, Lay-Ekuakille A (2018) Multispectral medical image fusion scheme based on hybrid contourlet and shearlet transform domains. Rev Sci Instrum 89(8):084301 Google Scholar
- Cai W, Ning X, Zhou G, Bai X, Jiang Y, Li W, Qian P (2022) A novel hyperspectral image classification model using bole convolution with three-directions attention mechanism: Small sample and unbalanced learning. IEEE Transactions on Geoscience and Remote Sensing
- Ch M, Riaz MM, Iltaf N, Ghafoor A, Sadiq MA (2019) Magnetic resonance and computed tomography image fusion using saliency map and cross bilateral filter. Signal, Image and Video Processing 13(6):1157–1164 Google Scholar
- Chai P, Luo X, Zhang Z (2017) Image fusion using quaternion wavelet transform and multiple features. IEEE access 5:6724–6734 Google Scholar
- Chang L, Feng X, Zhu X, Zhang R, He R, Xu C (2019) Ct and mri image fusion based on multiscale decomposition method and hybrid approach. IET Image Process 13(1):83–88 Google Scholar
- Chao Z, Kim D, Kim H-J (2018) Multi-modality image fusion based on enhanced fuzzy radial basis function neural networks. Physica Medica 48:11–20 Google Scholar
- Chavan SS, Mahajan A, Talbar SN, Desai S, Thakur M, D’cruz A (2017) Nonsubsampled rotated complex wavelet transform (nsrcxwt) for medical image fusion related to clinical aspects in neurocysticercosis. Comput Biol Med 81:64–78 Google Scholar
- Chen C-I (2017) Fusion of pet and mr brain images based on ihs and log-gabor transforms. IEEE Sensors J 17(21):6995–7010 Google Scholar
- Chen J, Zhang L, Lu L, Li Q, Hu M, Yang X (2021) A novel medical image fusion method based on rolling guidance filtering. Internet of Things 14:100172 Google Scholar
- Chinnadurai P, Duran C, Al-Jabbari O, Saleh WKA, Lumsden A, Bismuth J (2016) Value of c-arm cone beam computed tomography image fusion in maximizing the versatility of endovascular robotics. Annals of vascular surgery 30:138–148 Google Scholar
- Daniel E (2018) Optimum wavelet-based homomorphic medical image fusion using hybrid genetic-grey wolf optimization algorithm. IEEE Sensors J 18(16):6804–6811 Google Scholar
- Daniel E, Anitha J, Kamaleshwaran K, Rani I (2017) Optimum spectrum mask based medical image fusion using gray wolf optimization. Biomed Signal Process Control 34:36–43 Google Scholar
- Das M, Gupta D, Radeva P, Bakde AM (2020) Nsst domain ct-mr neurological image fusion using optimised biologically inspired neural network. IET Image Process 14(16):4291–4305 Google Scholar
- Das M, Gupta D, Radeva P, Bakde AM (2021) Optimized ct-mr neurological image fusion framework using biologically inspired spiking neural model in hybrid l1–l0 layer decomposition domain. Biomedical Signal Processing and Control 68:102535 Google Scholar
- Das M, Gupta D, Radeva P, Bakde AM (2021) Multi-scale decomposition-based ct-mr neurological image fusion using optimized bio-inspired spiking neural model with meta-heuristic optimization. Int J Imaging Syst Technol 31(4):2170–2188 Google Scholar
- Devanna H, Kumar G, Prasad G (2019) A spatio-frequency orientational energy based medical image fusion using non-sub sampled contourlet transform. Cluster Computing 22(5):11193–11205 Google Scholar
- Dhuli R, Polinati S (2020) Structural and functional medical image fusion using an adaptive fourier analysis. Multimedia Tools Appl 79(33):23645–23668 Google Scholar
- Ding Z, Zhou D, Li H, Hou R, Liu Y (2021) Siamese networks and multi-scale local extrema scheme for multimodal brain medical image fusion. Biomed Signal Process Control 68:102697 Google Scholar
- Ding Z, Zhou D, Nie R, Hou R, Liu Y (2020) Brain medical image fusion based on dual-branch cnns in nsst domain. BioMed Research International 2020
- Dinh P-H (2021) A novel approach based on three-scale image decomposition and marine predators algorithm for multi-modal medical image fusion. Biomed Signal Process Control 67:102536 Google Scholar
- Dinh P-H (2021) Multi-modal medical image fusion based on equilibrium optimizer algorithm and local energy functions. Appl Intell 51(11):8416–8431 Google Scholar
- Dinh P-H (2021) A novel approach based on grasshopper optimization algorithm for medical image fusion. Expert Systems with Applications 171:114576 Google Scholar
- Dinh P-H (2021) Combining gabor energy with equilibrium optimizer algorithm for multi-modality medical image fusion. Biomed Signal Process Control 68:102696 Google Scholar
- Diwakar M, Singh P, Shankar A Multi-modal medical image fusion framework using co-occurrence filter and local extrema in nsst domain. Biomedical Signal Processing and Control 68:102788
- Du J, Li W (2020) Two-scale image decomposition based image fusion using structure tensor. Int J Imaging Syst Technol 30(2):271–284 Google Scholar
- Du J, Li W, Lu K, Xiao B (2016) An overview of multi-modal medical image fusion. Neurocomputing 215:3–20 Google Scholar
- Du J, Li W, Xiao B (2018) Fusion of anatomical and functional images using parallel saliency features. Inf Sci 430:567–576 Google Scholar
- Du J, Li W, Tan H (2019) Intrinsic image decomposition-based grey and pseudo-color medical image fusion. IEEE Access 7:56443–56456 Google Scholar
- Du J, Li W, Tan H (2020) Three-layer medical image fusion with tensor-based features. Inf Sci 525:93–108 MathSciNetGoogle Scholar
- Duan C, Wang S, Huang Q, Wen T, Zhu C, Xu Y (2019) Feature level mri fusion based on 3d dual tree compactly supported shearlet transform. J Vis Commun Image Represent 60:319–327 Google Scholar
- Duan J, Mao S, Jin J, Zhou Z, Chen L, Chen CP (2021) A novel ga-based optimized approach for regional multimodal medical image fusion with superpixel segmentation. IEEE Access 9:96353–96366 Google Scholar
- Easley G, Labate D, Lim W-Q (2008) Sparse directional image representations using the discrete shearlet transform. Appl Comput Harmon Anal 25(1):25–46 MathSciNetGoogle Scholar
- El-Hoseny HM, Abd El-Rahman W, El-Rabaie E-SM, Abd El-Samie FE, Faragallah OS (2018) An efficient dt-cwt medical image fusion system based on modified central force optimization and histogram matching. Infrared Phys Technol 94:223–231 Google Scholar
- El-Hoseny HM, Abd El-Rahman W, El-Shafai W, El-Banby GM, El-Rabaie E-SM, Abd El-Samie FE, Faragallah OS, Mahmoud KR (2019) Efficient multi-scale non-sub-sampled shearlet fusion system based on modified central force optimization and contrast enhancement. Infrared Phys Technol 102:102975 Google Scholar
- El-Hoseny HM, El Kareh ZZ, Mohamed WA, El Banby GM, Mahmoud KR, Faragallah OS, El-Rabaie S, El-Madbouly E, El-Samie A, Fathi E (2019) An optimal wavelet-based multi-modality medical image fusion approach based on modified central force optimization and histogram matching. Multimedia Tools Appl 78(18):26373–26397 Google Scholar
- Faragallah OS, El-Hoseny H, El-Shafai W, Abd El-Rahman W, El-Sayed HS, El-Rabaie E-SM, Abd El-Samie FE, Geweid, G.G.: A comprehensive survey analysis for present solutions of medical image fusion and future directions. IEEE Access 9: 11358–11371
- Fu J, Li W, Du J, Xiao B (2020) Multimodal medical image fusion via laplacian pyramid and convolutional neural network reconstruction with local gradient energy strategy. Comput Biol Med 126:104048 Google Scholar
- Fu J, Li W, Du J, Huang Y (2021) A multiscale residual pyramid attention network for medical image fusion. Biomed Signal Process Control 66:102488 Google Scholar
- Fu J, Li W, Ouyang A, He B (2021) Multimodal biomedical image fusion method via rolling guidance filter and deep convolutional neural networks. Optik 237:166726 Google Scholar
- Fu J, Li W, Du J, Xu L (2021) Dsagan: A generative adversarial network based on dual-stream attention mechanism for anatomical and functional image fusion. Inf Sci 576:484–506 MathSciNetGoogle Scholar
- Gai D, Shen X, Cheng H, Chen H (2019) Medical image fusion via pcnn based on edge preservation and improved sparse representation in nsst domain. IEEE Access 7:85413–85429 Google Scholar
- Gao Y, Ma S, Liu J, Liu Y, Zhang X (2021) Fusion of medical images based on salient features extraction by pso optimized fuzzy logic in nsst domain. Biomed Signal Process Control 69:102852 Google Scholar
- Geng P, Sun X, Liu J (2017) Adopting quaternion wavelet transform to fuse multi-modal medical images. J Med Biol Eng 37(2):230–239 Google Scholar
- Ghassemian H (2016) A review of remote sensing image fusion methods. Inf Fusion 32:75–89 Google Scholar
- Goyal S, Singh V, Rani A, Yadav N (2020) Fprsgf denoised non-subsampled shearlet transform-based image fusion using sparse representation. SIViP 14(4):719–726 Google Scholar
- Goyal B, Lepcha DC, Dogra A, Bhateja V, Lay-Ekuakille A (2021) Measurement and analysis of multi-modal image fusion metrics based on structure awareness using domain transform filtering. Measurement 182:109663 Google Scholar
- Guo K, Li X, Zang H, Fan T (2020) Multi-modal medical image fusion based on fusionnet in yiq color space. Entropy 22(12):1423 MathSciNetGoogle Scholar
- Guo K, Li X, Hu X, Liu J, Fan T (2021) Hahn-pcnn-cnn: an end-to-end multi-modal brain medical image fusion framework useful for clinical diagnosis. BMC Medical Imaging 21(1):1–22 Google Scholar
- Gupta D (2018) Nonsubsampled shearlet domain fusion techniques for ct-mr neurological images using improved biological inspired neural model. Biocybernetics Biomed Eng 38(2):262–274 Google Scholar
- He K, Gong J, Xie L, Zhang X, Xu D (2021) Regions preserving edge enhancement for multisensor-based medical image fusion. IEEE Trans Instrum Meas 70:1–13 Google Scholar
- Hermessi H, Mourali O, Zagrouba E (2018) Convolutional neural network-based multimodal image fusion via similarity learning in the shearlet domain. Neural Comput & Applic 30(7):2029–2045 Google Scholar
- Hermessi H, Mourali O, Zagrouba E (2021) Multimodal medical image fusion review: Theoretical background and recent advances. Signal Process 183:108036 Google Scholar
- Hou R, Zhou D, Nie R, Liu D, Ruan X (2019) Brain ct and mri medical image fusion using convolutional neural networks and a dual-channel spiking cortical model. Med Biol Eng Comput 57(4):887–900 Google Scholar
- Hu Q, Hu S, Zhang F (2020) Multi-modality medical image fusion based on separable dictionary learning and gabor filtering. Signal Process Image Commun 83:115758 Google Scholar
- Hu Q, Hu S, Zhang F (2021) Multi-modality image fusion combining sparse representation with guidance filtering. Soft Comput 25(6):4393–4407 Google Scholar
- Huang C, Tian G, Lan Y, Peng Y, Ng EYK, Hao Y, Cheng Y, Che W (2019) A new pulse coupled neural network (pcnn) for brain medical image fusion empowered by shuffled frog leaping algorithm. Front Neurosci 13:210 Google Scholar
- Huang J, Le Z, Ma Y, Fan F, Zhang H, Yang L (2020) Mgmdcgan: Medical image fusion using multi-generator multi-discriminator conditional generative adversarial network. IEEE Access 8:55145–55157 Google Scholar
- Huang Y, Li W, Du J (2021) Anatomical-functional image fusion based on deep convolution neural networks in local laplacian pyramid domain. Int J Imaging Syst Technol 31(3):1246–1264 Google Scholar
- Huang X, Zhang B, Zhang X, Tang M, Miao Q, Li T, Jia G (2021) Application of u-net based multiparameter magnetic resonance image fusion in the diagnosis of prostate cancer. IEEE Access 9:33756–33768 Google Scholar
- Huang B, Yang F, Yin M, Mo X, Zhong C (2020) A review of multimodal medical image fusion techniques. Computational and mathematical methods in medicine 2020
- Jabason E, Ahmad MO, Swamy M (2019) Multimodal neuroimaging fusion in nonsubsampled shearlet domain using location-scale distribution by maximizing the high frequency subband energy. IEEE Access 7:97865–97886 Google Scholar
- Jiang W, Yang X, Wu W, Liu K, Ahmad A, Sangaiah AK, Jeon G (2018) Medical images fusion by using weighted least squares filter and sparse representation. Comput Electr Eng 67:252–266 Google Scholar
- Jiang J, Feng X, Hu Z, Hu X, Liu F, Huang H (2021) Medical image fusion using transfer learning and l-bfgs optimization algorithm. International Journal of Imaging Systems and Technology 31(4):2003–2013 Google Scholar
- Jiang Y, Ma Y (2020) Application of hybrid particle swarm and ant colony optimization algorithms to obtain the optimum homomorphic wavelet image fusion: introduction. Annals of Translational Medicine 8(22)
- Jin X, Chen G, Hou J, Jiang Q, Zhou D, Yao S (2018) Multimodal sensor medical image fusion based on nonsubsampled shearlet transform and s-pcnns in hsv space. Signal Process 153:379–395 Google Scholar
- Jin X, Jiang Q, Chu X, Lang X, Yao S, Li K, Zhou W (2019) Brain medical image fusion using l2-norm-based features and fuzzy-weighted measurements in 2-d littlewood-paley ewt domain. IEEE Trans Instrum Meas 69(8):5900–5913 Google Scholar
- Kang J, Lu W, Zhang W (2020) Fusion of brain pet and mri images using tissue-aware conditional generative adversarial network with joint loss. IEEE Access 8:6368–6378 Google Scholar
- Kar MK, Ravichandran G, Elangovan P, Nath MK (2019) Analysis of diagnostic features from fundus image using multiscale wavelet decomposition. ICIC Express Lett Part B: Appl 10(2):75–184 Google Scholar
- Kaur M, Singh D (2021) Multi-modality medical image fusion technique using multi-objective differential evolution based deep neural networks. J Ambient Intell Humanized Comput 12(2):2483–2493 Google Scholar
- Kavitha S, Thyagharajan K (2017) Efficient dwt-based fusion techniques using genetic algorithm for optimal parameter estimation. Soft Comput 21(12):3307–3316 Google Scholar
- Khare A, Khare M, Srivastava R (2021) Shearlet transform based technique for image fusion using median fusion rule. Multimedia Tools Appl 80(8):11491–11522 Google Scholar
- Kitchenham B, Charters S (2007) Guidelines for performing systematic literature reviews in software engineering. Keele University and Durham University Joint Report
- Kong W, Miao Q, Lei Y (2018) Multimodal sensor medical image fusion based on local difference in non-subsampled domain. IEEE Transactions on Instrumentation and Measurement 68(4):938–951 Google Scholar
- Kong W, Chen Y, Lei Y (2021) Medical image fusion using guided filter random walks and spatial frequency in framelet domain. Signal Process 181:107921 Google Scholar
- Kumar KV, Sathish A (2021) A comparative study of various multimodal medical image fusion techniques–a review. In: 2021 Seventh International Conference on Bio Signals, Images, and Instrumentation (ICBSII), pp. 1–6. IEEE
- Kumar P, Diwakar M (2021) A novel approach for multimodality medical image fusion over secure environment. Trans Emerg Telecommun Technol 32(2):3985 Google Scholar
- Li L, Ma H (2021) Pulse coupled neural network-based multimodal medical image fusion via guided filtering and wseml in nsct domain. Entropy 23(5):591 MathSciNetGoogle Scholar
- Li X, Zhao J (2021) A novel multi-modal medical image fusion algorithm. J Ambient Intell Humanized Comput 12(2):1995–2002 MathSciNetGoogle Scholar
- Li W, Xu X, Du J (2018) Multimodal sensor medical image fusion based on mutual-structure for joint filtering using sparse representation. Int J Imaging Syst Technol 28(1):3–14 Google Scholar
- Li W, Zhao J, Xiao B (2018) Multimodal medical image fusion by cloud model theory. SIViP 12(3):437–444 Google Scholar
- Li W, Jia L, Du J (2019) Multi-modal sensor medical image fusion based on multiple salient features with guided image filter. IEEE Access 7:173019–173033 Google Scholar
- Li Y, Lv Z, Zhao J, Pan Z (2019) Improving performance of medical image fusion using histogram, dictionary learning and sparse representation. Multimedia Tools Appl 78(24):34459–34482 Google Scholar
- Li X, Zhang X, Ding M (2019) A sum-modified-laplacian and sparse representation based multimodal medical image fusion in laplacian pyramid domain. Med Biol Eng Comput 57(10):2265–2275 Google Scholar
- Li B, Peng H, Luo X, Wang J, Song X, Pérez-Jiménez MJ, Riscos-Núñez A (2021) Medical image fusion method based on coupled neural p systems in nonsubsampled shearlet transform domain. Int J Neural Syst 31(01):2050050 Google Scholar
- Li X, Zhou F, Tan H, Zhang W, Zhao C (2021) Multimodal medical image fusion based on joint bilateral filter and local gradient energy. Information Sciences 569:s302-325 MathSciNetGoogle Scholar
- Li Q, Wang W, Chen G, Zhao D (2021) Medical image fusion using segment graph filter and sparse representation. Comput Biol Med 131:104239 Google Scholar
- Li W, Lin Q, Wang K, Cai K (2021) Improving medical image fusion method using fuzzy entropy and nonsubsampling contourlet transform. Int J Imaging Syst Technol 31(1):204–214 Google Scholar
- Li B, Peng H, Wang J (2021) A novel fusion method based on dynamic threshold neural p systems and nonsubsampled contourlet transform for multi-modality medical images. Signal Process 178:107793 Google Scholar
- Liu X, Mei W, Du H (2017) Structure tensor and nonsubsampled shearlet transform based algorithm for ct and mri image fusion. Neurocomputing 235:131–139 Google Scholar
- Liu X, Mei W, Du H (2018) Detail-enhanced multimodality medical image fusion based on gradient minimization smoothing filter and shearing filter. Med Biol Eng Comput 56(9):1565–1578 Google Scholar
- Liu X, Mei W, Du H (2018) Multi-modality medical image fusion based on image decomposition framework and nonsubsampled shearlet transform. Biomed Signal Process Control 40:343–350 Google Scholar
- Liu Y, Chen X, Ward RK, Wang ZJ (2019) Medical image fusion via convolutional sparsity based morphological component analysis. IEEE Signal Process Lett 26(3):485–489 Google Scholar
- Liu Z, Song Y, Sheng VS, Xu C, Maere C, Xue K, Yang K (2019) Mri and pet image fusion using the nonparametric density model and the theory of variable-weight. Comput Methods Prog Biomed 175:73–82 Google Scholar
- Liu Y, Zhou D, Nie R, Hou R, Ding Z, Guo Y, Zhou J (2020) Robust spiking cortical model and total-variational decomposition for multimodal medical image fusion. Biomedical Signal Processing and Control 61:101996 Google Scholar
- Liu Y, Wang L, Cheng J, Li C, Chen X (2020) Multi-focus image fusion: A survey of the state of the art. Inf Fusion 64:71–91 Google Scholar
- Liu Y, Zhang C, Li C, Cheng J, Zhang Y, Xu H, Song T, Zhao L, Chen X (2020) A practical pet/ct data visualization method with dual-threshold pet colorization and image fusion. Comput Biol Med 126:104050 Google Scholar
- Liu Y, Hou R, Zhou D, Nie R, Ding Z, Guo Y, Zhao L (2021) Multimodal medical image fusion based on the spectral total variation and local structural patch measurement. Int J Imaging Syst Technol 31(1):391–411 Google Scholar
- Li W, Wang K, Cai K (2019) Medical image fusion based on saliency and adaptive similarity judgment. Personal and Ubiquitous Computing, 1–7
- Lou, X., Feng, X.: Multimodal medical image fusion based on multiple latent low-rank representation. Computational and Mathematical Methods in Medicine 2021 (2021)
- Ma J, Ma Y, Li C (2019) Infrared and visible image fusion methods and applications: A survey. Inf Fusion 45:153–178 Google Scholar
- Manchanda M, Sharma R (2018) An improved multimodal medical image fusion algorithm based on fuzzy transform. J Vis Commun Image Represent 51:76–94 Google Scholar
- Maqsood S, Javed U (2020) Multi-modal medical image fusion based on two-scale image decomposition and sparse representation. Biomedical Signal Process Control 57:101810 Google Scholar
- Meng L, Guo X, Li H (2019) Mri/ct fusion based on latent low rank representation and gradient transfer. Biomed Signal Process Control 53:101536 Google Scholar
- Miao Y, Chunyu N, Yazhuo X (2021) Brain medical image fusion scheme based on shuffled frog-leaping algorithm and adaptive pulse-coupled neural network. IET Image Process 15(6):1203–1209 Google Scholar
- Muzammil S, Maqsood S, Haider S (2020) DamaševičiusR (2020) CSID: A Novel Multimodal Image Fusion Algorithm for Enhanced Clinical Diagnosis. Diagnostics 10:904 Google Scholar
- Na Y, Zhao L, Yang Y, Ren M (2018) Guided filter-based images fusion algorithm for ct and mri medical images. IET Image Process 12(1):138–148 Google Scholar
- Nair RR, Singh T (2019) Multi-sensor medical image fusion using pyramid-based dwt: a multi-resolution approach. IET Image Process 13(9):1447–1459 Google Scholar
- Nair RR, Singh T (2021) Mamif: multimodal adaptive medical image fusion based on b-spline registration and non-subsampled shearlet transform. Multimedia Tools Appl 80(12):19079–19105 Google Scholar
- Nair RR, Singh T (2021) An optimal registration on shearlet domain with novel weighted energy fusion for multi-modal medical images. Optik 225:165742 Google Scholar
- Nair RR, Singh T, Sankar R, Gunndu K (2021) Multi-modal medical image fusion using lmf-gan-a maximum parameter infusion technique. J Intell Fuzzy Syst 41(5):5375–5386 Google Scholar
- Nath MK, Dandapat S (2012) Differential entropy in wavelet sub-band for assessment of glaucoma. Int J Imaging Syst Technol 22(3):161–165 Google Scholar
- Nath MK, Dandapat S (2013) Multiscale ica for fundus image analysis. Int J Imaging Syst Technol 23(4):327–337 Google Scholar
- Ouerghi H, Mourali O, Zagrouba E (2018) Non-subsampled shearlet transform based mri and pet brain image fusion using simplified pulse coupled neural network and weight local features in yiq colour space. IET Image Process 12(10):1873–1880 Google Scholar
- Padmavathi K, Asha C, Maya VK (2020) A novel medical image fusion by combining tv-l1 decomposed textures based on adaptive weighting scheme. Engineering Science and Technology, an International Journal 23(1):225–239 Google Scholar
- Palkar B, Mishra D (2019) Fusion of multi-modal lumbar spine images using kekre’s hybrid wavelet transform. IET Image Process 13(12):2271–2280 Google Scholar
- Panigrahy C, Seal A, Mahato NK (2020) Mri and spect image fusion using a weighted parameter adaptive dual channel pcnn. IEEE Signal Processing Letters 27:690–694 Google Scholar
- Paramanandham N, Rajendiran K (2018) Infrared and visible image fusion using discrete cosine transform and swarm intelligence for surveillance applications. Infrared Phys Technol 88:13–22 Google Scholar
- Parvathy VS, Pothiraj S (2020) Multi-modality medical image fusion using hybridization of binary crow search optimization. Health Care Manag Rev 23(4):661–669 Google Scholar
- Patil HV, Shirbahadurkar SD (2018) Fwfusion: Fuzzy whale fusion model for mri multimodal image fusion. Sādhanā 43(3):1–16 MathSciNetGoogle Scholar
- Pei C, Fan K, Wang W (2020) Two-scale multimodal medical image fusion based on guided filtering and sparse representation. IEEE Access 8:140216–140233 Google Scholar
- Polinati S, Dhuli R (2020) Multimodal medical image fusion using empirical wavelet decomposition and local energy maxima. Optik 205:163947 Google Scholar
- Prakash O, Park CM, Khare A, Jeon M, Gwak J (2019) Multiscale fusion of multimodal medical images using lifting scheme based biorthogonal wavelet transform. Optik 182:995–1014 Google Scholar
- Qi S, Ning X, Yang G, Zhang L, Long P, Cai W, Li W (2021) Review of multi-view 3d object recognition methods based on deep learning. Displays 69:102053 Google Scholar
- Rajalingam B, Priya R, Bhavani R (2019) Hybrid multimodal medical image fusion using combination of transform techniques for disease analysis. Procedia Comput Sci 152:150–157 Google Scholar
- Rajalingam B, Al-Turjman F, Santhoshkumar R, Rajesh M (2020) Intelligent multimodal medical image fusion with deep guided filtering. Multimedia Systems, 1–15
- Ramlal SD, Sachdeva J, Ahuja CK, Khandelwal N (2019) An improved multimodal medical image fusion scheme based on hybrid combination of nonsubsampled contourlet transform and stationary wavelet transform. International Journal of Imaging Systems and Technology 292):146–160
- Ramlal SD, Sachdeva J, Ahuja CK, Khandelwal N (2018) Multimodal medical image fusion using non-subsampled shearlet transform and pulse coupled neural network incorporated with morphological gradient. SIViP 12(8):1479–1487 Google Scholar
- Reena Benjamin J, Jayasree T (2018) Improved medical image fusion based on cascaded pca and shift invariant wavelet transforms. Int J CARS 13(2):229–240 Google Scholar
- Sandhya S, Senthil Kumar M, Karthikeyan L (2019) A hybrid fusion of multimodal medical images for the enhancement of visual quality in medical diagnosis. In: Computer Aided Intervention and Diagnostics in Clinical and Medical Images, pp. 61–70. Springer
- Shahdoosti HR, Mehrabi A (2018) Multimodal image fusion using sparse representation classification in tetrolet domain. Dig Signal Process 79:9–22 MathSciNetGoogle Scholar
- Shahdoosti HR, Mehrabi A (2018) Mri and pet image fusion using structure tensor and dual ripplet-ii transform. Multimedia Tools Appl 77(17):22649–22670 Google Scholar
- Shehanaz S, Daniel E, Guntur SR, Satrasupalli S (2021) Optimum weighted multimodal medical image fusion using particle swarm optimization. Optik 231:166413 Google Scholar
- Shibu DS, Priyadharsini SS (2021) Multi scale decomposition based medical image fusion using convolutional neural network and sparse representation. Biomed Signal Process Control 69:102789 Google Scholar
- Singh S, Anand RS (2018) Ripplet domain fusion approach for ct and mr medical image information. Biomed Signal Process Control 46:281–292 Google Scholar
- Singh S, Anand RS (2019) Multimodal medical image sensor fusion model using sparse k-svd dictionary learning in nonsubsampled shearlet domain. IEEE Trans Instrum Meas 69(2):593–607 Google Scholar
- Singh S, Anand RS (2019) Multimodal medical image fusion using hybrid layer decomposition with cnn-based feature mapping and structural clustering. IEEE Trans Instrum Meas 69(6):3855–3865 Google Scholar
- Singh S, Gupta D (2020) Detail enhanced feature-level medical image fusion in decorrelating decomposition domain. IEEE Trans Instrum Meas 70:1–9 Google Scholar
- Singh S, Gupta D (2021) Multistage multimodal medical image fusion model using feature-adaptive pulse coupled neural network. Int J Imaging Syst Technol 31(2):981–1001 Google Scholar
- Soundrapandiyan R, Karuppiah M, Kumari S, Kumar Tyagi S, Wu F, Jung K-H (2017) An efficient dwt and intuitionistic fuzzy based multimodality medical image fusion. Int J Imaging Syst Technol 27(2):118–132 Google Scholar
- Srivastava A, Bhateja V, Moin A (2017) Combination of pca and contourlets for multispectral image fusion. In: Proceedings of the International Conference on Data Engineering and Communication Technology, pp. 577–585. Springer
- Subbiah Parvathy V, Pothiraj S, Sampson J (2020) A novel approach in multimodality medical image fusion using optimal shearlet and deep learning. Int J Imaging Syst Technol 30(4):847–859 Google Scholar
- Sufyan A, Imran M, Shah SA, Shahwani H, Wadood AA (2022) A novel multimodality anatomical image fusion method based on contrast and structure extraction. Int J Imaging Syst Technol 32(1):324–342 Google Scholar
- Sunderlin Shibu D, Suja Priyadharsini S (2021) Multimodal medical image fusion using l0 gradient smoothing with sparse representation. Int J Imaging Syst Technol 31(4):2249–2266 Google Scholar
- Tan W, Thitøn W, Xiang P, Zhou H (2021) Multi-modal brain image fusion based on multi-level edge-preserving filtering. Biomed Signal Process Control 64:102280 Google Scholar
- Tang L, Qian J, Li L, Hu J, Wu X (2017) Multimodal medical image fusion based on discrete tchebichef moments and pulse coupled neural network. Int J Imaging Syst Technol 27(1):57–65 Google Scholar
- Tang L, Tian C, Xu K (2018) Igm-based perceptual multimodal medical image fusion using free energy motivated adaptive pcnn. Int J Imaging Syst Technol 28(2):99–105 Google Scholar
- Tannaz A, Mousa S, Sabalan D, Masoud P Fusion of multimodal medical images using nonsubsampled shearlet transform and particle swarm optimization. Multidimensional Systems and Signal Processing 31( 1):269–287
- Tan W, Tiwari P, Pandey HM, Moreira C, Jaiswal AK (2020) Multimodal medical image fusion algorithm in the era of big data. Neural Computing and Applications, 1–21
- Tan L, YuX X (2019) Medical image fusion based on fast finite shearlet transform and sparse representation. Computational and mathematical methods in medicine 2019
- Tawfik N, Elnemr HA, Fakhr M, Dessouky MI, El-Samie A, Fathi E (2021) Survey study of multimodality medical image fusion methods. Multimedia Tools Appl 80(4):6369–6396 Google Scholar
- Tawfik N, Elnemr HA, Fakhr M, Dessouky MI, El-Samie A, Fathi E (2021) Hybrid pixel-feature fusion system for multimodal medical images. J Ambient Intell Humanized Comput 12(6):6001–6018 Google Scholar
- Tirupal T, Chandra Mohan B, Srinivas Kumar S (2019) Multimodal medical image fusion based on yager’s intuitionistic fuzzy sets. Iran J Fuzzy Syst 16(1):33–48 MathSciNetGoogle Scholar
- Ullah H, Ullah B, Wu L, Abdalla FY, Ren G, Zhao Y (2020) Multi-modality medical images fusion based on local-features fuzzy sets and novel sum-modified-laplacian in non-subsampled shearlet transform domain. Biomed Signal Process Control 57:101724 Google Scholar
- Vanitha K, Satyanarayana D, Prasad MG (2021) Multi-modal medical image fusion algorithm based on spatial frequency motivated pa-pcnn in the nsst domain. Curr Med Imaging 17(5):634–643 Google Scholar
- Venkatrao PH, Damodar SS (2018) Hwfusion: Holoentropy and sp-whale optimisation-based fusion model for magnetic resonance imaging multimodal image fusion. IET Image Process 12(4):572–581 Google Scholar
- Vishwakarma A, Bhuyan MK, Iwahori Y (2018) Non-subsampled shearlet transform-based image fusion using modified weighted saliency and local difference. Multimedia Tools Appl 77(24):32013–32040 Google Scholar
- Wang Q, Yang X (2020) An efficient fusion algorithm combining feature extraction and variational optimization for ct and mr images. J Appl Clin Med Phys 21(6):139–150 Google Scholar
- Wang L, Shi C, Lin S, Qin P, Wang Y (2020) Convolutional sparse representation and local density peak clustering for medical image fusion. Int J Pattern Recognit Artif Intell 34(07):2057003 Google Scholar
- Wang K, Zheng M, Wei H, Qi G, Li Y (2020) Multi-modality medical image fusion using convolutional neural network and contrast pyramid. Sensors 20(8):2169 Google Scholar
- Wang Z, Cui Z, Zhu Y (2020) Multi-modal medical image fusion by laplacian pyramid and adaptive sparse representation. Comput Biol Med 123:103823 Google Scholar
- Wang G, Li W, Huang Y (2021) Medical image fusion based on hybrid three-layer decomposition model and nuclear norm. Comput Biol Med 129:104179 Google Scholar
- Wang L, Zhang J, Liu Y, Mi J, Zhang J (2021) Multimodal medical image fusion based on gabor representation combination of multi-cnn and fuzzy neural network. IEEE Access 9:67634–67647 Google Scholar
- Wang Z, Li X, Duan H, Su Y, Zhang X, Guan X (2021) Medical image fusion based on convolutional neural networks and non-subsampled contourlet transform. Expert Systems with Applications 171:114574 Google Scholar
- Xia K, Yin H, Wang J (2019) A novel improved deep convolutional neural network model for medical image fusion. Clus Comput 22(1):1515–1527 Google Scholar
- Xia J, Lu Y, Tan L (2020) Research of multimodal medical image fusion based on parameter-adaptive pulse-coupled neural network and convolutional sparse representation. Computational and Mathematical Methods in Medicine 2020
- Xu, Z., Xiang, W., Zhu, S., Zeng, R., Marquez-Chin, C., Chen, Z., Chen, X., Liu, B., Li, J.: Latlrr-fcns: latent low-rank representation with fully convolutional networks for medical image fusion. Frontiers in Neuroscience, 1387 (2021)
- Xu H, Ma J (2021) Emfusion: An unsupervised enhanced medical image fusion network. Inf Fusion 76:177–186 Google Scholar
- Yadav SP, Yadav S (2020) Image fusion using hybrid methods in multimodality medical images. Med Biol Eng Comput 58(4):669–687 Google Scholar
- Yang Y, Wu J, Huang S, Fang Y, Lin P, Que Y (2018) Multimodal medical image fusion based on fuzzy discrimination with structural patch decomposition. IEEE J Biomed Health inform 23(4):1647–1660 Google Scholar
- Yang Z, Chen Y, Le Z, Fan F, Pan E (2019) Multi-source medical image fusion based on wasserstein generative adversarial networks. IEEE Access 7:175947–175958 Google Scholar
- Yang Y, Cao S, Huang S, Wan W (2020) Multimodal medical image fusion based on weighted local energy matching measurement and improved spatial frequency. IEEE Trans Instrum Meas 70:1–16 Google Scholar
- Yin M, Liu X, Liu Y, Chen X (2018) Medical image fusion with parameter-adaptive pulse coupled neural network in nonsubsampled shearlet transform domain. IEEE Trans Instrum Meas 68(1):49–64 Google Scholar
- Zhang X, Yan H (2021) Medical image fusion and noise suppression with fractional-order total variation and multi-scale decomposition. IET Image Process 15(8):1688–1701 Google Scholar
- Zhang Z, Cui J, Luo X, You Q (2020) Statistical correlative model in the multimodal fusion of brain images. Int J Imaging Syst Technol 30(4):1066–1079 Google Scholar
- Zhang Z, Xi X, Luo X, Jiang Y, Dong J, Wu X (2021) Multimodal image fusion based on global-regional-local rule in nsst domain. Multimedia Tools Appl 80(2):2847–2873 Google Scholar
- Zhang H, Yan W, Zhang C, Wang L (2021) Research on image fusion algorithm based on nsst frequency division and improved lscn. Mob Netw Appl 26(5):1960–1970 Google Scholar
- Zhang L, Zhang Y, Ma S, Yang F (2021) Ct and mri image fusion algorithm based on hybrid l0l1 layer decomposing and two-dimensional variation transform. Biomed Signal Process Control 70:103024 Google Scholar
- Zhang S, Li X, Zhu R, Zhang X, Wang Z, Zhang S (2021) Medical image fusion algorithm based on l0 gradient minimization for ct and mri. Multimedia Tools Appl 80(14):21135–21164 Google Scholar
- Zhao M, Peng Y (2021) A multi-module medical image fusion method based on non-subsampled shear wave transformation and convolutional neural network. Sensing and Imaging 22(1):1–16 MathSciNetGoogle Scholar
- Zhao F, Xu G, Zhao W (2019) Ct and mr image fusion based on adaptive structure decomposition. IEEE Access 7:44002–44009 Google Scholar
- Zhou T, Lu H, Hu F, Shi H, Qiu S, Wang H (2021) A new robust adaptive fusion method for double-modality medical image pet/ct. BioMed Research International 2021
- Zhu Z, Yin H, Chai Y, Li Y, Qi G (2018) A novel multi-modality image fusion method based on image decomposition and sparse representation. Inf Sci 432:516–529 MathSciNetGoogle Scholar
- Zhu Z, Zheng M, Qi G, Wang D, Xiang Y (2019) A phase congruency and local laplacian energy based multi-modality medical image fusion method in nsct domain. IEEE Access 7:20811–20824 Google Scholar
- Zhu R, Li X, Zhang X, Ma M (2020) Mri and ct medical image fusion based on synchronized-anisotropic diffusion model. IEEE Access 8:91336–91350 Google Scholar
- Zong J, Qiu T (2017) Medical image fusion based on sparse representation of classified image patches. Biomed Signal ProcessControl 34:195–205 Google Scholar
Funding
The authors did not receive support from any organization for the submitted work.
No funding was received to assist with the preparation of this manuscript.
No funding was received for conducting this study.